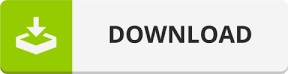
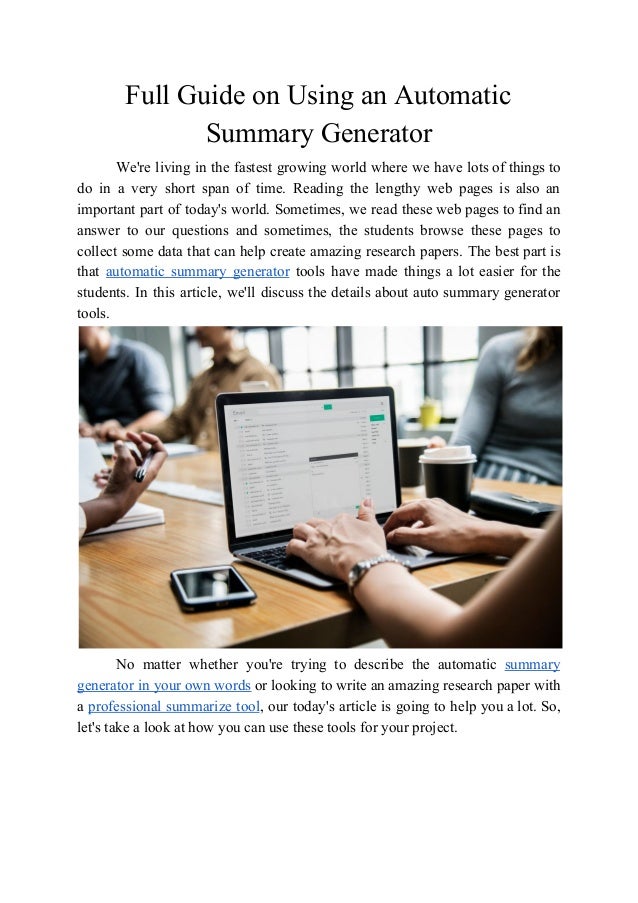
Once the PDF is imported, the PDFgear chatbot will automatically generate the suggested questions, and you can use prompts like “summarize the PDF” to summarize PDF and other types of documents in seconds. PDFgear ushers a way to read PDFs with ChatGPT. Powered by the GPT-3.5 model, PDFgear works both as an AI chatbot and PDF summarizer.

#BOOK SUMMARY GENERATOR SOFTWARE#
PDFgear Chatbot is a ground-breaking feature from the free PDF editor software PDFgear Desktop that allows users to summarize text and information in large PDF documents in simple words and sentences, which makes it a better PDF summarizer tool.
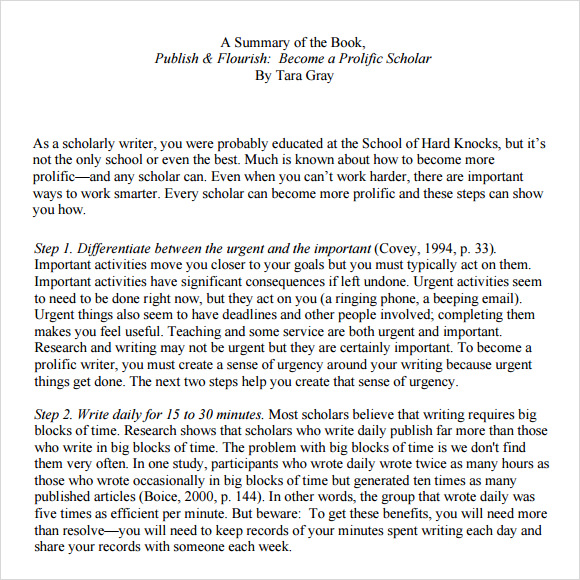
The online paraphraser and summarizer that supports adjusting the summary lengthĬonvert text from various sources into interactive flashcards Immediately summarize article URL or text online without signing up The web-based tool to chat with PDF and summarize PDFĪ multi-language PDF summarizer that keeps a summary history The omnipotent AI that condenses text under specific prompts
#BOOK SUMMARY GENERATOR OFFLINE#
Top 7 AI Summarizers Compared SummarizersĬompletely free offline PDF chatbot and summarizer on Windows and Mac Some of them already have successful use cases in education.Īll introduced tools have been tested using a sample PDF essay with over 70, 000 words.Īdditionally, to help improve your studying and working efficiency, I’d like to suggest that you check our list of the best free PDF editors and free scanner apps as well. On this page, you’ll discover the 7 best AI-powered tools that can summarize, extract important information from, and rewrite books, essays, textbooks, and more. Luckily, today with the information in this post, you can still catch up. Going forward, we are researching better ways to assist humans in evaluating model behavior, with the goal of finding techniques that scale to aligning artificial general intelligence.If you’re still manually summarizing your articles and papers, you’re tremendously falling behind your peers who’re using AI. Our progress on book summarization is the first large-scale empirical work on scaling alignment techniques. In this case, to evaluate book summaries we empower humans with individual chapter summaries written by our model, which saves them time when evaluating these summaries relative to reading the source text. Our current approach to this problem is to empower humans to evaluate machine learning model outputs using assistance from other models. Therefore we want our ability to evaluate our models to increase as their capabilities increase. This makes it harder to detect subtle problems in model outputs that could lead to negative consequences when these models are deployed. This work is part of our ongoing research into aligning advanced AI systems, which is key to our mission. As we train our models to do increasingly complex tasks, making informed evaluations of the models’ outputs will become increasingly difficult for humans. Our method can be used to summarize books of unbounded length, unrestricted by the context length of the transformer models we use.See for yourself on our summary explorer! For example, you can trace to find where in the original text certain events from the summary happen. It is easier to trace the summary-writing process.Decomposition allows humans to evaluate model summaries more quickly by using summaries of smaller parts of the book rather than reading the source text.Compared to an end-to-end training procedure, recursive task decomposition has the following advantages: In this case we break up summarizing a long piece of text into summarizing several shorter pieces. To address this problem, we additionally make use of recursive task decomposition: we procedurally break up a difficult task into easier ones. But judging summaries of entire books takes a lot of effort to do directly since a human would need to read the entire book, which takes many hours. In the past we found that training a model with reinforcement learning from human feedback helped align model summaries with human preferences on short posts and articles. Large pretrained models aren’t very good at summarization. Consider the task of summarizing a piece of text.
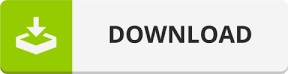